Our Research Exchange series features the work of researchers across The National Archives in which they discuss their new discoveries and their work’s potential for impact. The purpose of the series is to highlight and demystify the research we do and open it up to new ideas and discussion.
This month and next, to coincide with our upcoming Annual Digital Lecture, we will be publishing a series of blogs focusing on different aspects of digital research happening at The National Archives.
Our staff will be writing and reflecting on their research, discussing topics such as using AI in archives, using 3D Virtual Tech to access heritage collections, archiving citizen research projects, and much more.
In this blog, Jenny Bunn discusses AI in archives.
Jenny writes:
This blog post offers a personal reflection on the subject of artificial intelligence (AI) in the archives. It is based on a presentation given at DCDC 2022.
Whenever I am asked to define AI, I choose to do so in the terms in which it was originally defined – in a proposal for a summer workshop to be held at Dartmouth College in New Hampshire in 1956. Working in these terms, I define AI as a long-standing academic project to explore the hypothesis that ‘every aspect of learning or any other feature of intelligence can in principle be so precisely described that a machine can be made to simulate it.’ (see footnote 1)
This project is still very much ongoing and this hypothesis is still far from proven one way or the other. More certain though is that the knowledge and techniques developed through this exploration have found fruitful and profitable application in any number of real world contexts. As this has happened, new fields have emerged that seek to further this application into specific areas. For example, one of the first new fields to spin off from the quest for AI was that of robotics. This field has achieved considerable success in proving the hypothesis that one feature of intelligence that it is possible to describe, such that a machine can simulate it, is the ability to follow a clearly set out procedure, to get that is with the programme. Indeed machines can do so quite literally, such that they are able to do so arguably more slavishly and consistently than a human would ever be capable of. Because they can simulate this feature, they can and do perform routine and repetitive manual tasks, albeit under carefully controlled environmental conditions.
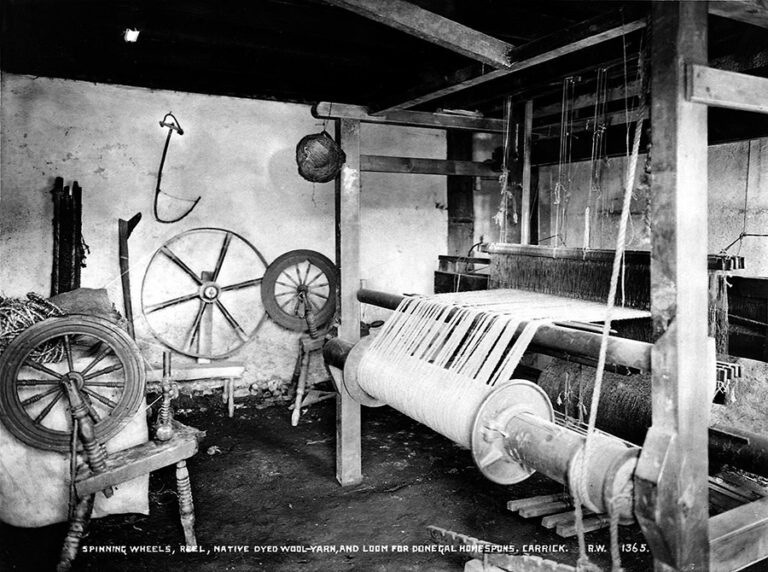
More recently, another spin-off from the exploration of AI has been the field of machine learning. The feature of intelligence that machine learning simulates is our ability to process data to iterate internal ‘mental’ models that allow us to make judgements or predictions based on similar data in the future. To some extent then this is learning by experience, but in the case of machine learning that experience is exceedingly limited in range. Even so however, the machine simulation of this feature has advanced to the extent that it already mimics the almost unconscious internalisation of the arrived at model, which leaves many experts unable to fully explain why and how they have come to those conclusions or by those insights. Then again, the simulation also mimics the well-known problems of reaching any conclusion purely on the basis (or experience) of data that is inaccurate, incomplete and/or unrepresentative in some important respect.
Training and refining these machine mental models takes a lot of time, a lot of energy and a lot of data, but models have now been created that do allow machines to simulate more advanced features of intelligence, such as the ability to parse natural language, or to recognise what objects appear in an image. As the phrase goes, fake it until you make it, but while the machines may now be able to fake it, it is still up to us what we make of it.
What then should we make of it? What then are we making of it? The recent report of the EuropeanaTech AI for GLAMs (Galleries, Libraries, Archives and Museums) Task Force offers a snapshot of how this part of the cultural heritage sector has applied AI so far, as well as where the GLAM interest lies in developing it further (footnote 2). Two of the areas where its application has already been found useful, and hence two of the purposes for which GLAMs are interested in developing AI, are knowledge extraction and (meta)data quality. Knowledge extraction – the uncovering of latent insights from data – is core to the GLAM mission, but it is also dependent on data quality.
Ensuring data quality has always been a resource-heavy enterprise. GLAM organisations have always added value (or quality) in at least two ways. Both through their material preservation and care of their collections, and their creation, extraction and curation of lots of additional intelligence, information or metadata that allows the relevant material to be found, understood and sourced from those collections when required. With the arrival of new digital ways of processing however, to be considered ‘quality’, all this material and information is now expected to be accessible in a new form which is amenable to that type of processing – we are entering the world of, so the shorthand goes, collections as data (footnote 3).
It is my observation therefore, that the main purpose for which AI is currently being applied within GLAM contexts is that of datafication – of turning collections into useable data, of spinning them into digital gold. It is through the application of machine learning that projects such as Transkribus have allowed for leaps forward in handwritten text recognition (footnote 4). This application then makes the resulting text amenable to another application of AI – that of natural language processing – and one project to experiment with this application was The Cybernetics Thought Collective project at the University of Illinois (footnote 5). Working on the digitised papers of leading cyberneticians, they used these techniques to generate metadata ‘automatically’ and there are many further examples of similar experiments (footnote 6).
Data-fying and increasing the computability and hence discoverability of the knowledge base held by cultural heritage institutions may well be a noble and worthwhile aim, but it is one that should be undertaken consciously. This consciousness will perhaps be easier to maintain if we try to frame future discussion of AI in the Archives as being less about AI and more about our application of it – the path GLAM organisations choose to follow with it.
Footnotes
- McCarthy J, Minsky M, Rochester, N and Shannon, C E, A Proposal for the Dartmouth Summer Research Project on Artificial Intelligence, 1955. Available at: dart564props.pdf (raysolomonoff.com)
- Europeana Network Association, AI in relation to GLAMs Task Force: Report and recommendations, 2021. Available at AI in relation to GLAMs | Europeana Pro
- Padilla, T, Allen, L, Frost, H, Potvin, S, Russey Roke, E and Varner, S, Final Report — Always Already Computational: Collections as Data (Version 1), 2019. Available at: https://doi.org/10.5281/zenodo.3152935
- Transkribus | AI-powered Handwritten Text Recognition (readcoop.eu)
- Anderson, B G, Prom, C J, Hutchinson, J A, Chandrashekhar, A, Michael, B, Udhani, S, Sammons, M, Dolski, A, Hamilton, K, Kaushik, S and Shrivastava, M, The Cybernetics Thought Collective: A History of Science and Technology Portal Project: White Paper, 2019. Available at: The Cybernetics Thought Collective: A History of Science and Technology Portal Project White Paper | IDEALS (illinois.edu)
- An early example of work in this area is Greenberg J, Spurgin, K and Crystal A, Final Report of the AMeGA (Automatic Metadata Generation Applications) Project, 2005. Available at: Functional Requirements for Automatic Metadata Generation Applications (loc.gov)